TL;DR
Santa Ana Biosciences is a precision immunology drug development company, leveraging multi-omics platforms and antibody engineering to deliver targeted therapies to patients with autoimmune and inflammatory diseases. They aim to develop safe, efficacious, and easy to deliver therapies.
2x Faster Decision Making
The computational biology team went serverless with pods, accelerating their decision making by 2x.
New Drug Targets Identified with 1 Million+ Cell Atlas
They collaboratively built a single-cell Atlas on the cloud integrating millions of cells from 300+ single-cell studies into R-based environments for analysis.
Successful IND Filings for the FDA
The team has an environment of version controlled data, code and pipelines. All changes are logged and easily traceable, a must-have for FDA submissions.
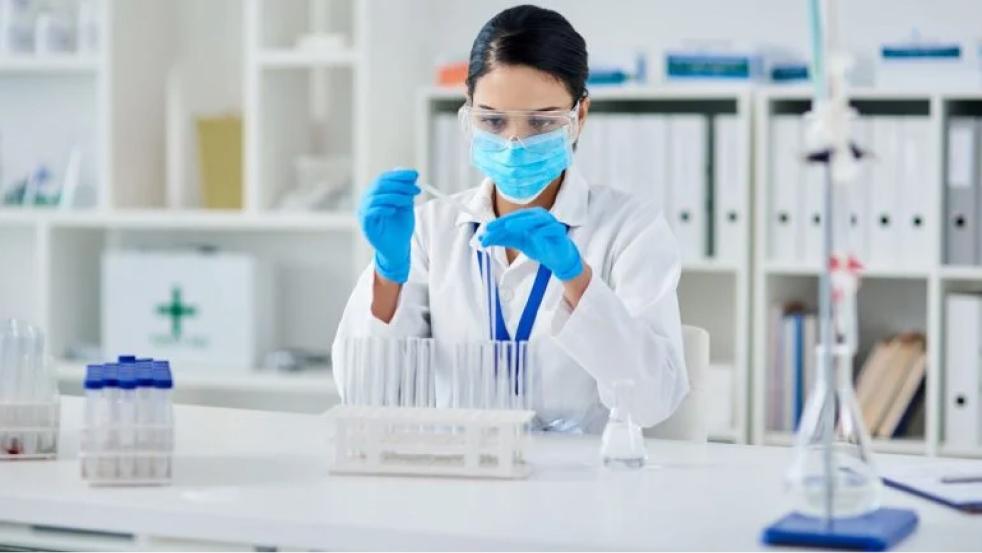
Santa Ana Bio's Story
Santa Ana is pioneering a new frontier in autoimmune disease treatments by harnessing the power of multi-omics and sophisticated data analysis. Their target diseases are broad within the autoimmune spectrum, and their mission is to introduce precision therapeutics grounded in deep insights from patient-derived data.
By collecting actual human patient tissues samples and sequencing them at single cell resolution, they gain deeper insights into which cell types, pathways, and targets matter order to develop better therapies.
The platform group at Santa Ana is a rag-tag team of drug hunters divided into several specialized teams:
Fundamentally, the platform team is responsible for supporting all of the programs with any question they have. Questions like:
- What are the pathways in this disease that really matter?
- What gene is critical to which cell types? (target nomination)
- How is this target affected by our products? (target validation)
- Can we target this gene or cell type in this way? (product concept validation)
- We have 50 diseases to treat, which ones make the most sense? (Indication prioritization)
Challenges
Building a data insight layer for these questions is challenging. Key challenges include data organization, scalability, and compute.
Data organization
Every dataset needs to live in a single place so that everyone agrees about what they're talking about,” says Danny, the Chief Technology Officer.
We have so much data, and we’re a small team, so making sure our processes keep everything clean, happy, and tidy is super important.”
Scalability
Every computational biologist was going to be doing their own data ops, to scale up machines, go to AWS, add GPUs, extend the storage, etc.”
To scale, the team started with a custom-grown AWS data platform. For primary processing, they purchased SevenBridges, and used AWS EC2 and Sagemaker for downstream collaborative analysis. However, these solutions proved expensive, inflexible, and time consuming to maintain.
We realized people were losing like two to three days a month, 15% of their time, just to Sagemaker ops.”
Decision making
These solutions often crashed or led to 20+ minute startup times, and this meant computational biologists would have to wait before starting new experiments. If something crashed, they would need to restart. This process led to slower R&D cycles and slower results to the board.
Solution
LatchBio enabled the transition from inefficient, isolated data management and analysis systems on AWS to a unified, scalable platform.
Latch Pods for Collaborative Computational Biology
The computational biology team migrated to Latch Pods to move more quickly and make faster decisions. Instead of isolated environments, they all began to share one single filesystem with access their own virtual machines, and were given the ability to flexibly scale machines up and down within minutes.
Pod resizing for flexible access to compute
Instead of wasting time managing infrastructure or waiting 20+ mins for instances to start up, Santa Ana used containerized pod environments which run much more efficiently, allowing the team to create pods for each experiment and run them in parallel, resizing them to add GPUs, 500 GiB of memory, and up to 16 TB of storage per experiment.
One platform to replace them all
The team eliminated needless spend on 3rd party data storage and NGS software vendors, saving $80,000 on software licenses and $24,000 on AWS.
Outcomes
Faster R&D cycles for the organization, leading to successful IND
We can just move so fast and answer so many questions. We move really, really, really, really quickly. And that's always what I've wanted. We fly, we don't have to think about it... This gains us credibility as a team, and we can help support the company better through faster responses and decision making”
Scaled up T-cell Atlas from 500,000 to millions of cells
Our existing T Cell Atlas was 500,000, and we decided to 10x this for a strategy... When you have a compbio team that's empowered to be able to just ask questions, move at scale, and not be limited by computational resources, it leads to faster insight.”
Saved 36 days a year per computational biologist
We estimated people were losing like two to three days a month, 15% of their time, just to Sage maker ops... Now all this time is spent on real analysis.”
Conclusion
Santa Ana bio is a cutting edge biotech team pioneering the future of immunotherapies. By adopting a modern tech stack, their computational biology team is able to move 2-3x faster, make better decisions, and accelerate their time to the clinic.