TL;DR
Gensaic is an MIT-founded team of phage and biological engineers working on solving therapeutic delivery. They rely heavily on next-generation sequencing and machine learning to identify tissue-targeting moieties for delivering therapeutics.
With Latch, Gensaic now is able to manage raw data and host bioinformatics pipelines and machine learning workflows in one central platform, accelerating their discovery process and enabling easier collaboration for everyone. This has resulted in:
Accelerated Evolution Screening by 1.5x
Gensaic accelerated the interpretation of large-scale data across evolution screens far more efficiently with Latch.
Flexibly scaling of ML and cloud compute workflows
Pods gave Gensaic flexibility around their compute resources and helped compartmentalize different analyses easily.
Increased access to data for experimentalists
“Latch made the experimental team much more close to the data side. Undoubtedly, this is going to help us progress our science.”
Gensaic’s Story
Tremendous improvements to patient health are constrained by therapeutic delivery. An explosion of promising therapeutic modalities (RNAi, ASOs, gene therapies, CRISPR) show great potential in cell culture — but consistently fail to deliver to specific organs or disease contexts. Delivering payloads in the body is a difficult biological engineering problem to solve.
Founded at MIT and based off of technology from the 2018 Nobel Prize in Chemistry, Gensaic is a team of phage and biological engineers working on solving in-vivo delivery. Their platform aims to deliver any gene to any cell or tissue.
“We're relying on what works in nature: it's biological diversity. Biological diversity is the foundation of how we discover how to solve delivery. Our thesis is that delivery is a multi-dimensional challenge... To address a multi-dimensional problem, you usually need high diversity in a very powerful screening platform.”
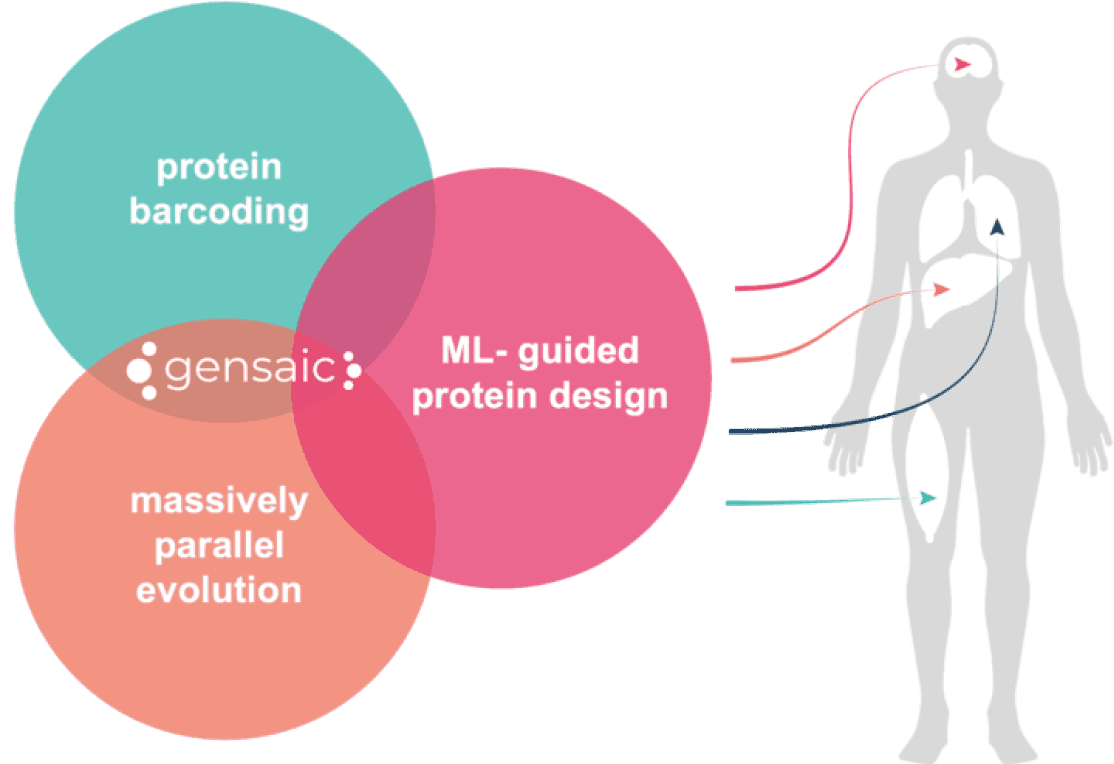
The team is revealing delivery mechanisms through three pillars:
- Protein barcoding technology: By mapping diverse protein scaffolds with nucleic acid barcodes, they can evolve proteins towards tissues of interest.
- Diverse screening contexts: A massively parallel evolution platform driven by various types of screening, including in vitro, cellular, and in vivo, to discover proteins that bind to target receptors. They then use NGS to measure which proteins mediate delivery of different payloads.
- Integrative and generative machine learning: The third pillar is using machine learning to integrate and understand datasets, and to employ generative models like variational auto-encoders for de-novo sequence generation. They then repeat steps 1-3 using the de-novo sequences.
The team is currently focusing on delivering gene therapies using phage-derived particles and other payloads like oligonucleotides.
Finding Adequate Computing
In R&D, Gensaic deals with massive genomics data which requires flexible, scalable computers to process. When Jonathan previously worked in an academic setting he had access to a large-scale computing cluster, memory, and file storage to do his research. He wanted this for his team at Gensaic:
I was looking for just a very simple thing: access to larger compute resources. I was looking literally for a seamless extension of my personal computer...”
He knew hiring someone to setup AWS was unncessesary, and decided to search for platforms that gave him flexible access to the compute he needed.
Latch Pods: Enabling Gensaic's Computational Platform
What really drew me to Latch was the idea of a Pod, because it is a very nice interface to kind of visualize a lot of very simple virtual machines. Not only could you kind of have flexibility around the resources you give to each machine, it also helped compartmentalize different analyses really easily”
With different screens coming through, I used each Pod as literally its own analysis for that dataset...almost a way to organize my own brain when I was doing analyses.”
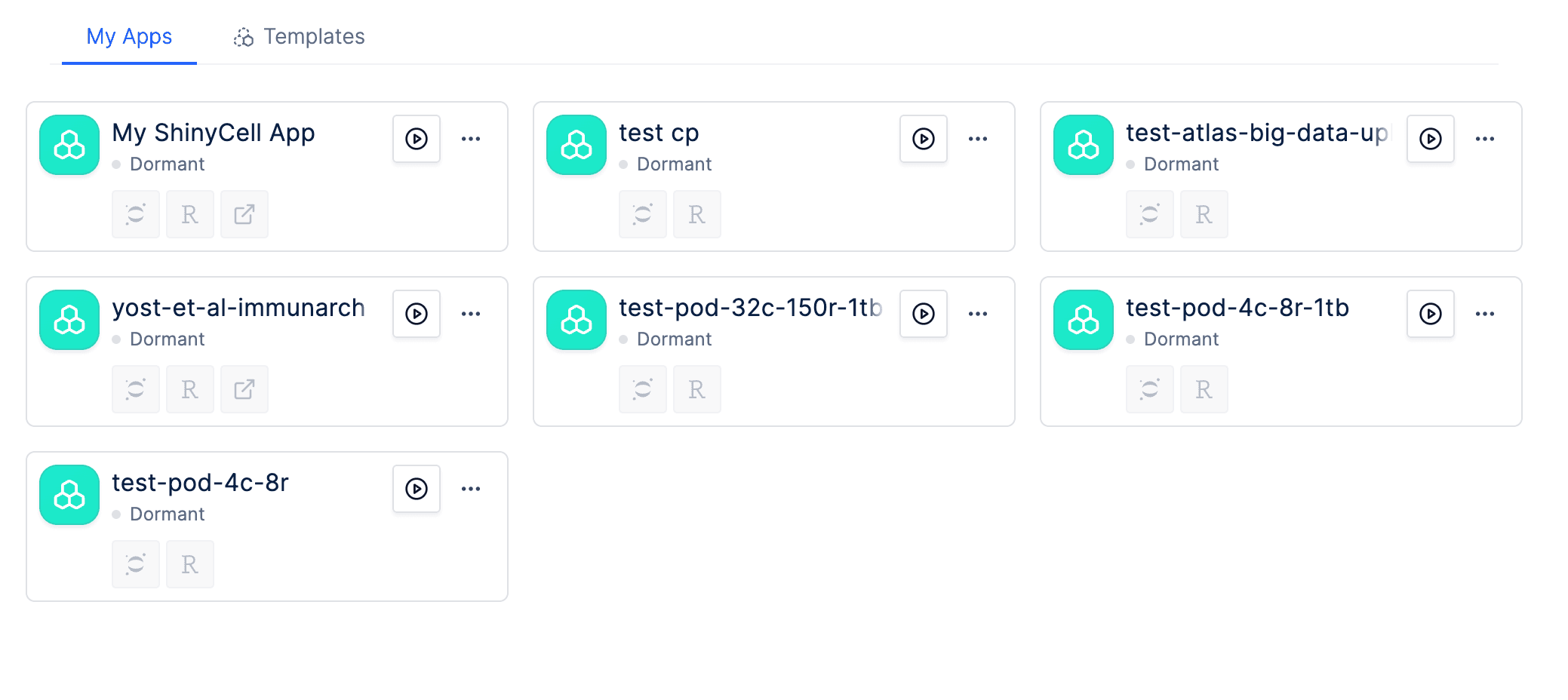
Using pods in tandem with the workflow... it has largely made the processing of our FastQ to Dataframe seamless.”
Gensaic now organizes raw data management, bioinformatics pipelines, and machine learning workflows in one central platform, accelerating their discovery process and enabling easier collaboration for everyone.
Going from our evolution screens, to sequencing, to interpretation of large-scale data across different screens is much more efficient with Latch.
Pods allow for others within our company who are not necessarily computationally focused to explore the data, side by side with with me... As a platform, it's really a shared space to not only analyze, but interpret data together.
I think it's an enabler of any company that needs to analyze data. If you work with large NGS datasets and need custom workflows, Latch is an ideal computational solution.”

Jonathan Hsu, Chief Technology Officer, Gensaic